The Challenge at Hand
Companies often require chatbots that excel in specific technical domains, such as medical advice, legal guidance, or customer support for a particular product. However, ChatGPT, while powerful, is not always equipped to handle these custom use cases. Therefore, the challenge lies in combining the power of ChatGPT with custom knowledge bases to create specialized chatbots that cater to unique business needs.

Potential Solutions: Weighing the Options
When it comes to customizing ChatGPT with a custom knowledge base, there are two primary approaches: fine-tuning and in-context learning.
Fine-tuning can result in higher performance for specific domains and is more suited for large-scale custom datasets. However, it requires access to the model's weights, more computational resources, and can be time-consuming and complex to implement.
On the other hand, in-context learning is quick and easy to implement, allowing for real-time adaptation to new information. Its main drawbacks are being limited by the context size (maximum of 4,000 tokens) and potentially requiring more context to achieve desired performance.
The Chosen Solution: In-Context Learning in Action
We choose in-context learning for this blog post, as it is a more accessible and straightforward approach to customizing ChatGPT with custom knowledge bases. This solution works by leveraging a vector database system built from a custom knowledge database. The core idea is to use similarity search to find the most relevant information from the custom database to include in the input context for ChatGPT, enabling it to provide more specialized responses.
Understanding Vector Databases
Vector databases are designed to store documents as high-dimensional vectors, which are multi-dimensional representations of words or phrases. This storage method enables efficient similarity searches, or "vector searches," which allow users to find relevant information without relying on specific keywords or metadata. This process returns similar or near-neighbor matches, providing a more comprehensive list of results. To store documents in this format, the database uses a process called embedding, which converts each word into a vector with hundreds or thousands of dimensions.

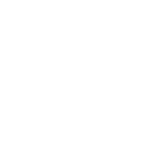